Inception-v4-Inception-ResNet
本课程主要以时间线为基础,详细讲解深度学习领域最重要的一些论文,目前的论文list如下:
Understanding the difficulty of training deep feedforward neural networks:关于模型初始化,激活函数等方面的讨论。
(ReLU)Deep Sparse Rectifier Neural Networks:关于经典激活函数ReLU的介绍。
(Dropout)Improving neural networks by preventing co-adaptation of feature detectors:关于深度学习中常用的抵抗过拟合技术Dropout的介绍。
(AlexNet)ImageNet Classification with Deep Convolutional Neural Networks:2012 ImageNet Challenge图像识别比赛冠军,深度学习在图像领域的经典开山之作。
(VGGNet)Very Deep Convolutional Networks for Large-Scale ImageRecognition:2014 ImageNet Challenge图像识别比赛亚军,广泛应用的一种图像识别模型。
(GoogleNet)Going deeper with convolutions:2014 ImageNet Challenge图像识别比赛冠军,谷歌提出的经典图像识别模型。
(BN)Batch Normalization: Accelerating Deep Network Training by Reducing Internal Covariate Shift:深度学习领域中常用的标准化策略。
(ResNet)Deep Residual Learning for Image Recognition:2015 ImageNet Challenge图像识别比赛冠军,2019年被应用了18000多次的经典深度学习论文。
(ResNet-v2)Identity Mappings in Deep Residual Networks:ResNet的改进版。
(Inception-v2/v3)Rethinking the Inception Architecture for Computer Vision:谷歌Inception系列的作品。
(Inception-v4/Inception-ResNet)Inception-v4, Inception-ResNet and the Impact of Residual Connections on Learning:谷歌Inception系列的作品。
(Group Convolution)Deep Roots:Improving CNN Efficiency with Hierarchical Filter Groups:组卷积介绍。
(ResNeXt)Aggregated Residual Transformations for Deep Neural Networks:2016 ImageNet Challenge图像识别比赛亚军。
(SENet)Squeeze-and-Excitation Networks:2017 ImageNet Challenge图像识别比赛冠军。
(GPT-3)Language Models are Few-Shot Learners:2020年5月由OpenAI发布,这是有史以来最大的NLP模型。
公开笔记对他人可见,有机会被管理员评为“优质笔记”
{{ noteEditor.content.length }}/2000
讲师收到你的提问会尽快为你解答。若选择公开提问,可以获得更多学员的帮助。
课程大纲
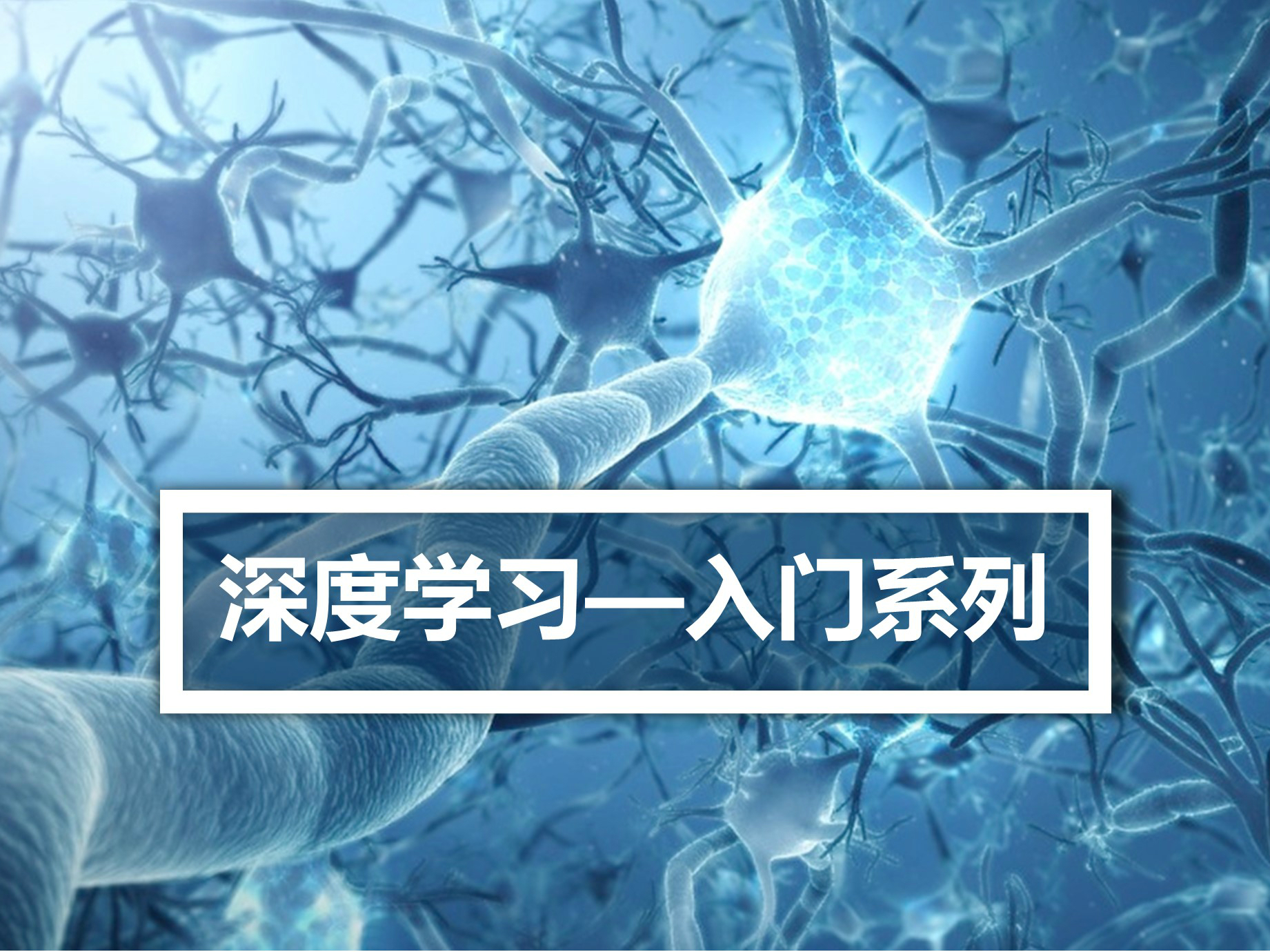
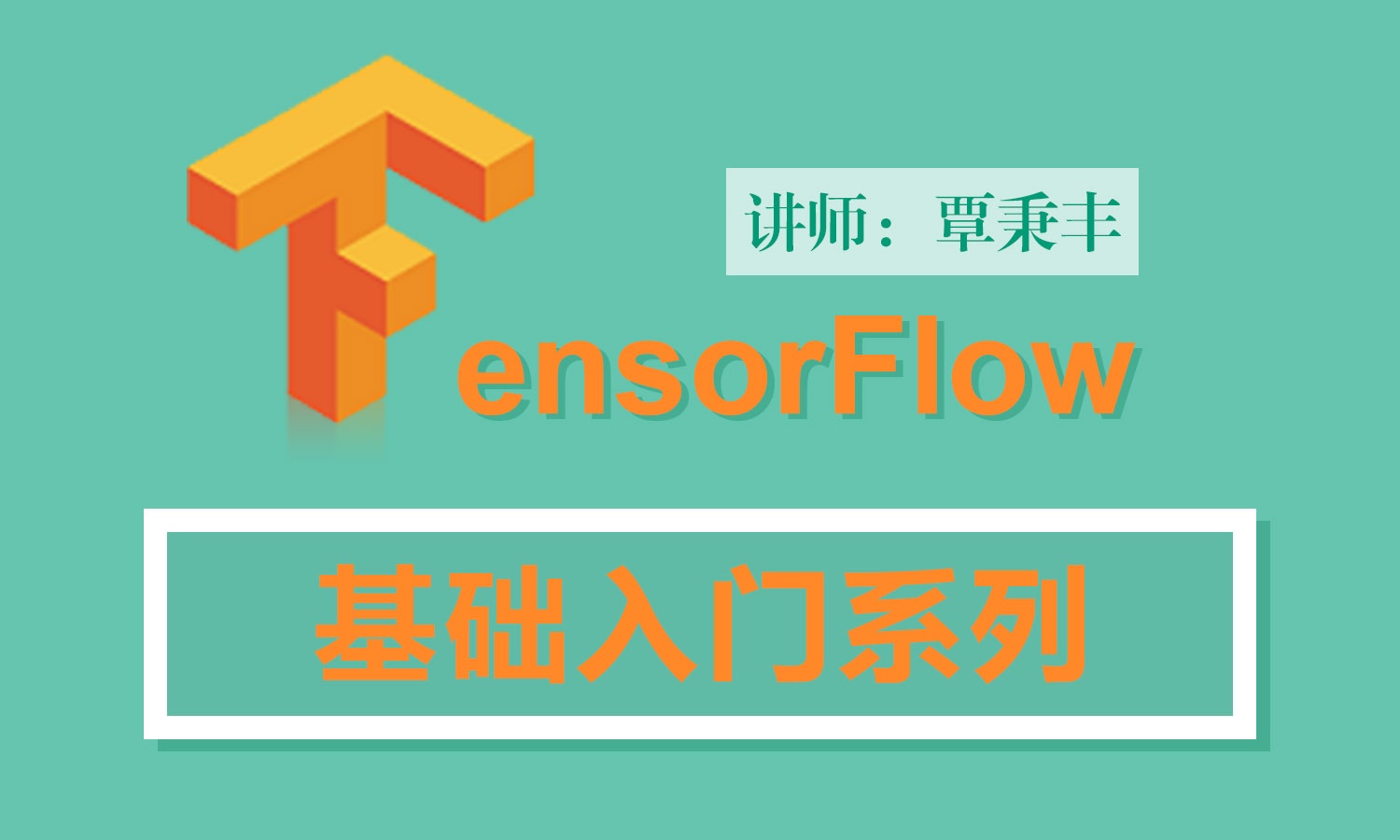
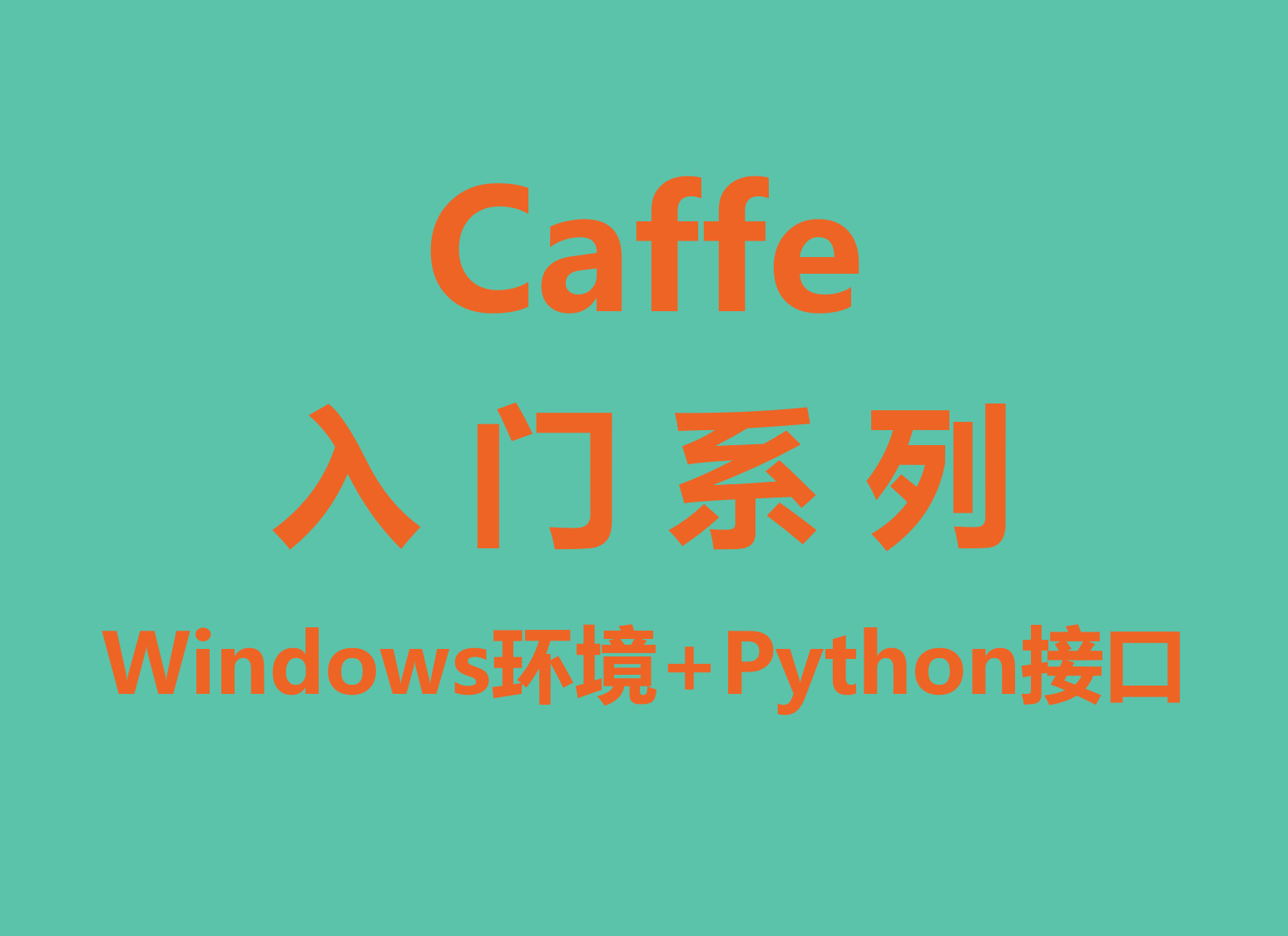
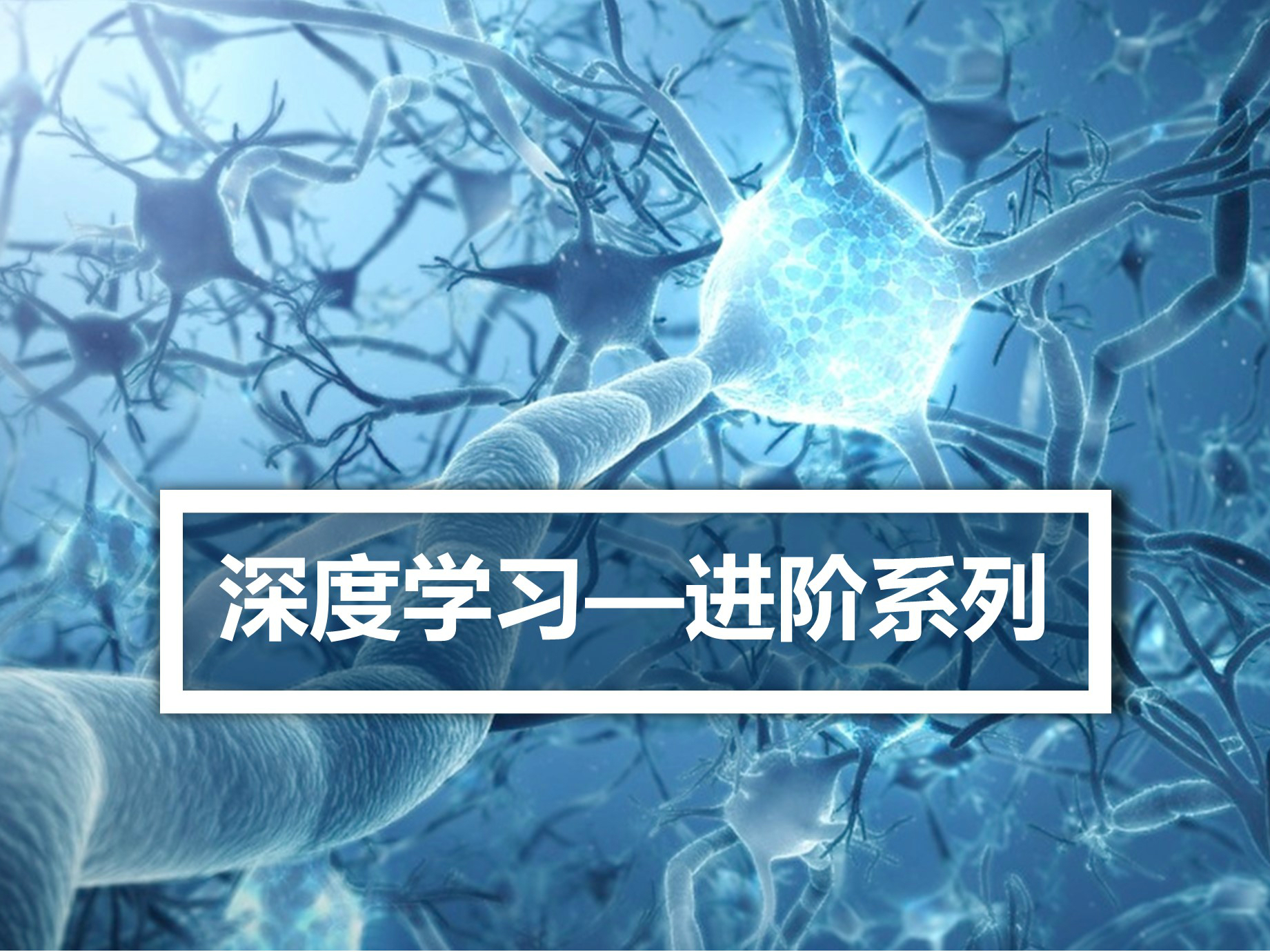
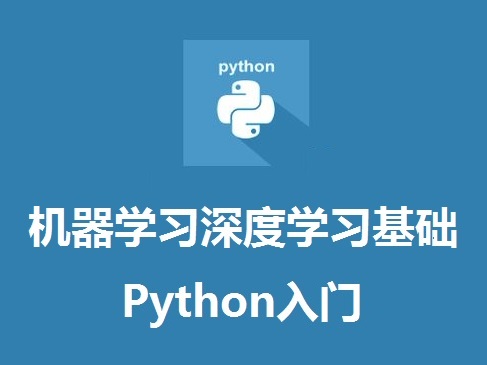
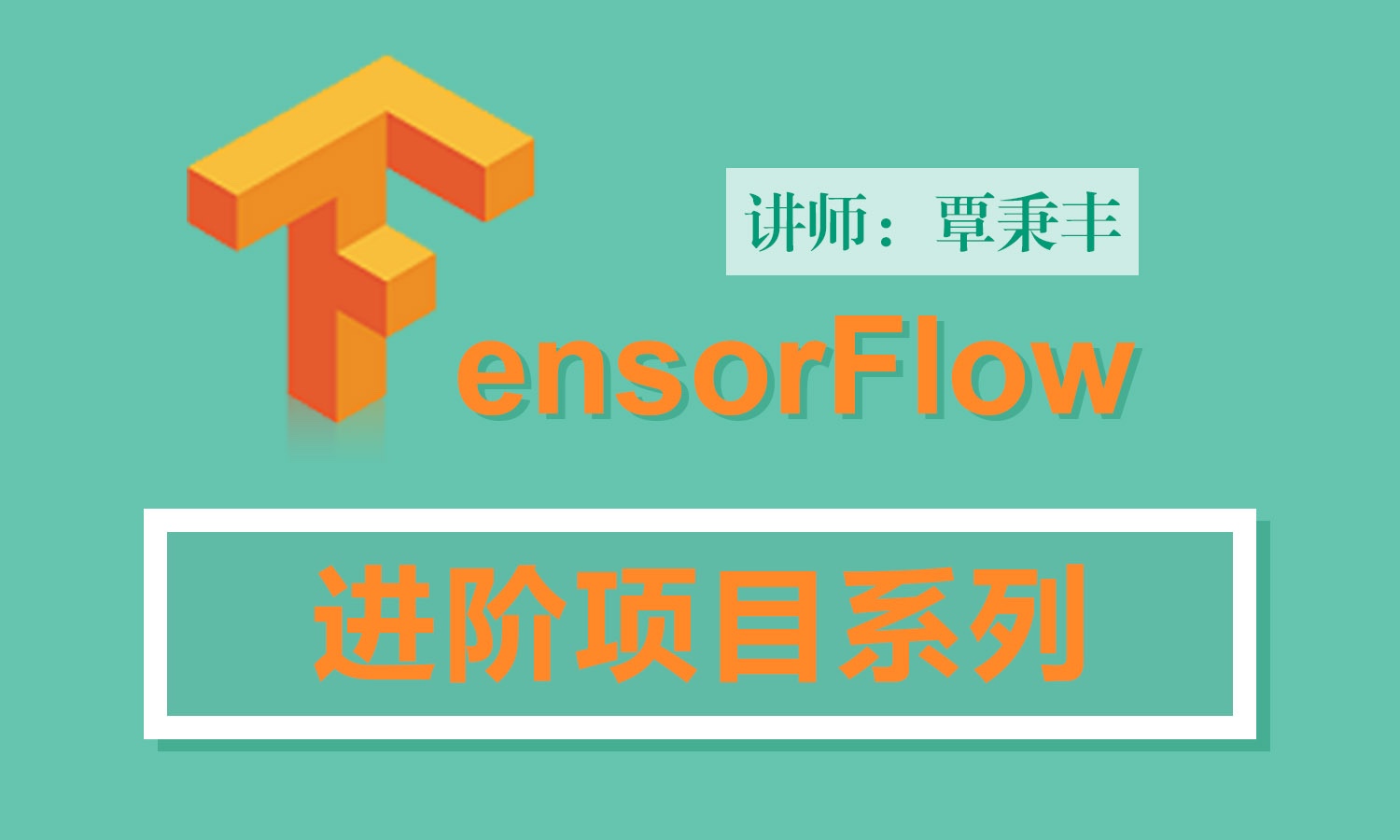
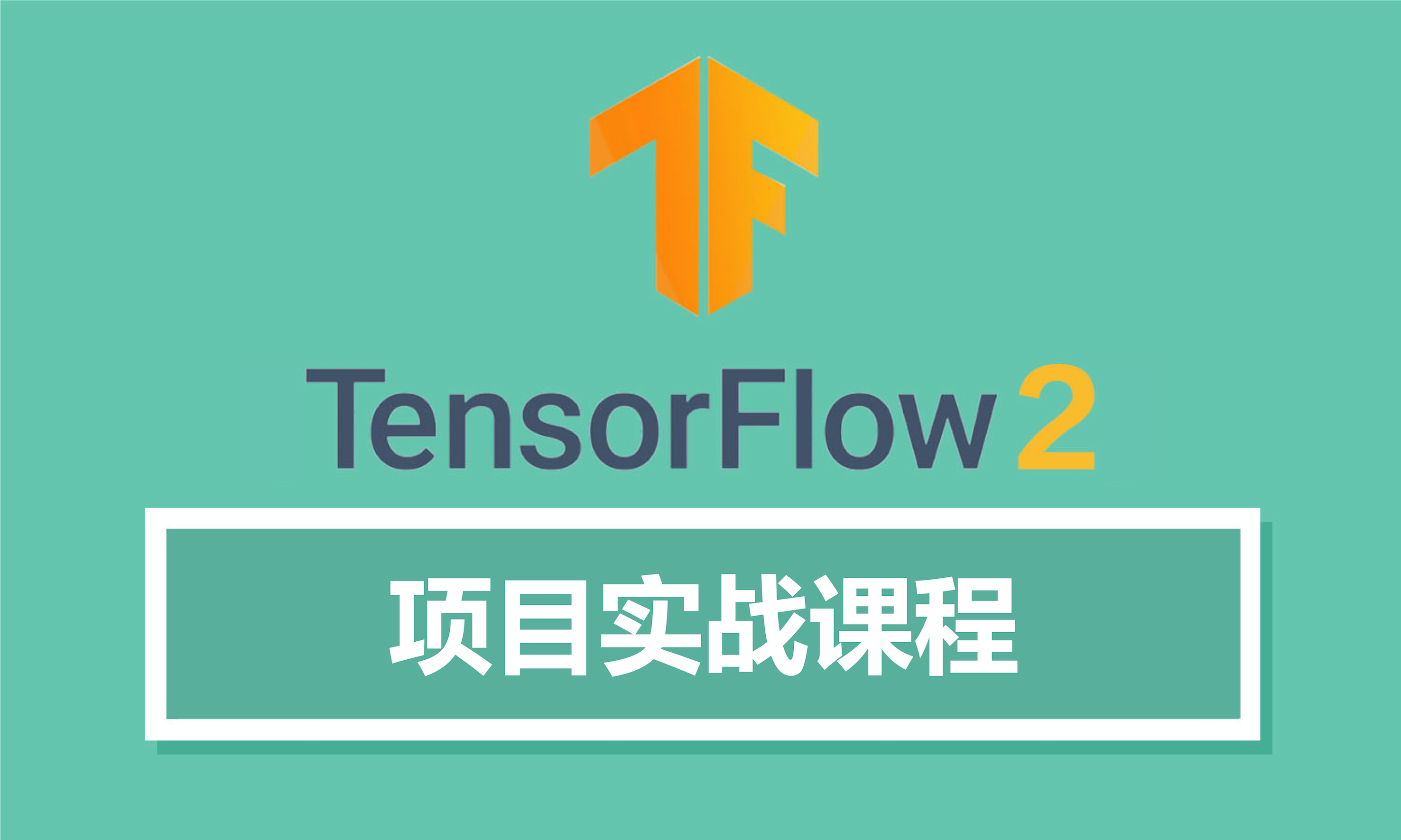
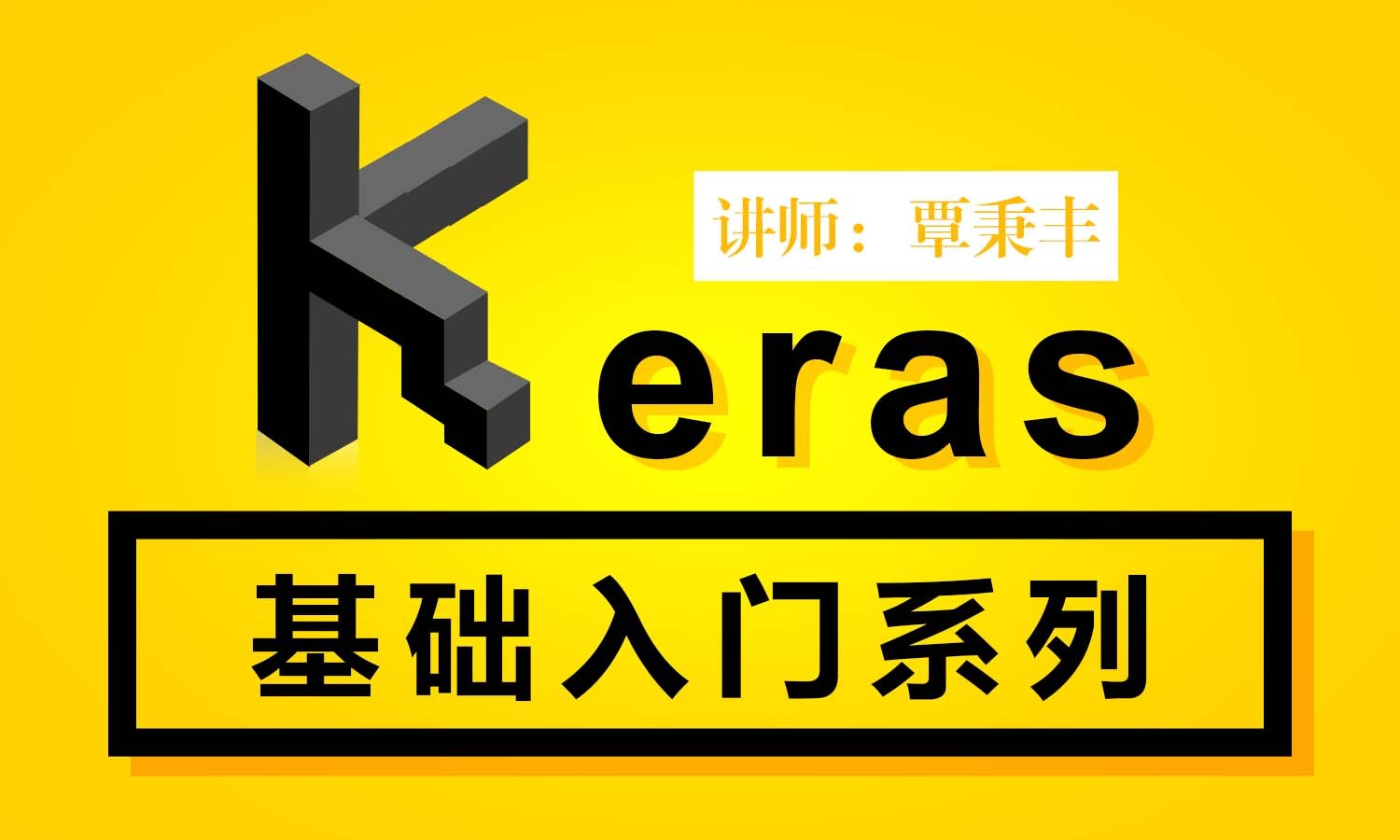